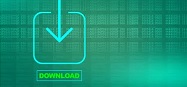
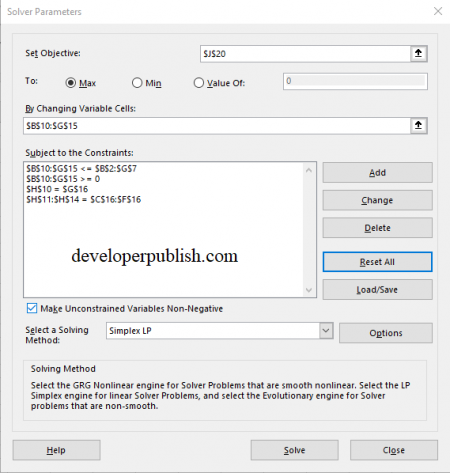

In particular, larger prediction errors should be associated with adjustments in subsequent decisions, which occur because prediction errors strengthen or weaken representations of winning and losing actions, respectively. Critically, these models suggest that reward prediction errors can guide decision-making by signaling the need to adjust future behavior. According to many reinforcement learning models, differences between expected and obtained reinforcements, or reward “prediction errors,” can be used to form and adjust associations between actions or stimuli and their ensuing reinforcements ( Sutton, 1992 Sutton and Barto, 1998 Montague and Berns, 2002). Recent research in neuroscience and computational modeling suggests that reinforcement learning theory provides a useful framework within which to study the neural mechanisms of reward-based learning and decision-making ( Schultz et al., 1997 Sutton and Barto, 1998 Dayan and Balleine, 2002 Montague and Berns, 2002 Camerer, 2003). These findings provide novel evidence that humans engage a reinforcement learning process to adjust representations of competing decision options. Furthermore, FRNs to decision outcomes were disproportionately larger over the motor cortex contralateral to the response hand that was used to make the decision. Consistent with predictions of a computational reinforcement learning model, we found that the magnitude of ERPs after losing to the computer opponent predicted whether subjects would change decision behavior on the subsequent trial. Analyses of ERP data focused on the feedback-related negativity (FRN), an outcome-locked potential thought to reflect a neural prediction error signal.

We recorded event-related brain potentials (ERPs) while subjects played a strategic economic game against a computer opponent to evaluate how neural responses to outcomes related to subsequent decision-making. In the present study, we tested the hypothesis that this flexibility emerges through a reinforcement learning process, in which reward prediction errors are used dynamically to adjust representations of decision options. Optimal behavior in a competitive world requires the flexibility to adapt decision strategies based on recent outcomes.
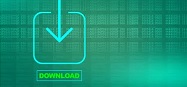